Abstract:
Target detection is a paramount task in remote sensing which aims to detect points of interest from a set of data. A crucial aspect attributed to the success of target detection methods is the representation of the data which goes into them. Consequentially, feature representation learning has been explored extensively in the literature [1, 2, 3, 4]. Discriminative feature learning approaches in the literature, however, have two common pitfalls. First, traditional approaches require vast quantities of precisely labeled groundtruth to guide training, which is typically difficult or impossible to acquire in practice. Second, current feature learning approaches which address label imprecision are limited in the features they can represent. In this work, the Multiple Instance Choquet Integral (MICI) [5] is explored as a nonlinear method for discriminative feature selection and fusion. Specifically, the MICI with a binary fuzzy measure was investigated as a method to select discriminative instance-level features. The MICI with a full measure was then explored as an instance-level classifier on the selected sources. The approaches explored learn solely from imprecisely annotated data. Experimental results on both synthetic and real-world remote sensing datasets indicate the utility of the MICI as an approach for down-selecting sources form a set of features. A MICI fusing the sources provided by the discriminative feature selection approach demonstrated competitive target detection
performance compared to class activation map methods in the literature.
Links: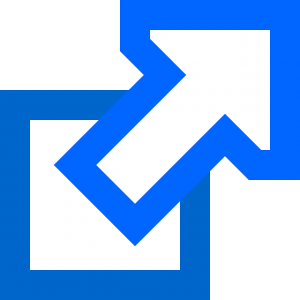
Citation:
C. McCurley, "Discriminative Feature Learning with Imprecise, Uncertain, and Ambiguous Data," Ph.D Thesis, Gainesville, FL, 2022.
@phdthesis{McCurley2022Thesis,
author = {Connor McCurley},
title = {Discriminative Feature Learning with Imprecise, Uncertain, and Ambiguous Data},
school = {Univ. of Florida},
year = {2022},
address = {Gainesville, FL},
}