Abstract:
Imprecise labels or label uncertainty are common problems in many real supervised and semi-supervised learning problems. However, most of the state-of-the-art supervised learning methods in the literature rely on accurate labels. Accurate labels are often either expensive, time-consuming, or even impossible to obtain in many real applications. There are many approaches in the literature that address imprecise and uncertain labels of various types. However, not all types of label uncertainty and imprecision are addressed. Furthermore, the overwhelming majority of methods that address label imprecision and uncertainty generally address only one form of imprecision/uncertainty, even when many problems have more than one type of imprecise label coexisting in a problem.
Multiple instance (MI) label is one type of imprecise label. MI label is a shared label for a set of instances, or ”bag”, instead of one instance. For example, in tree species classification from remotely sensed hyperspectral imagery, precise species class labels for each pixel are often difficult or expensive to obtain, as stated above. In comparison, an imprecise species label can be much easier to be assigned to a region (a bag) in the hyperspectral image, which can be a tree polygon, generated by an ecologist or tree delineation algorithm. The imprecise species label indicates the existence of the labeled species in the region as a form of subpixel, a pixel, or several pixels. However, this MI assumption, assuming the existence of accurate bag labels, may not hold in some applications. For instance, tree species labeling requires expertise in tree species knowledge, and sometimes relatives of a tree species are difficult to tell apart, thus the MI labels (species class label) for the bags (tree polygon) may be incorrect. In this case, another imprecise label, probabilistic label, can be introduced to incorporate the imprecision of MI labels. A probabilistic label is the confidence degree assigned to the original label as a way to show how probable the labeling by the ecologist is correct. In this case, a mixture of two types of imprecise labels, probabilistic labels and multiple instance labels, both present in the training set.
In this dissertation, different types of imprecise labels are defined and reviewed. A novel multi-imprecise label learning approach is proposed to address classification problems for real scenarios where probabilistic labels over the multiple instance bag-level labels present. In addition, the classes in the multiple instance problems are modeled by distributions (e.g. Gaussian Mixture Model) instead of learning prototypes to account for within-class variabilities. The classification approach proposed is applied to a variety of data sets with label uncertainty including hyperspectral data sets and medical imagery. Learning a distribution for each class of the imprecisely labeled data unveils the latent per-class distribution, not only improving the classification performance but also offering a way to visualize the variability of each class and deepen our understanding of the data, compared with other prototype-based or discriminative methods.
Links: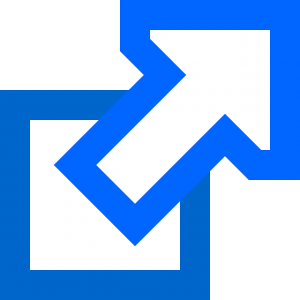
Citation:
S. Zou, “Classification with Multi-Imprecise Labels,” Ph.D. Thesis, Gainesville, FL, 2021.
@phdthesis{Zou2021Thesis,
author = {Sheng Zou},
title = {Classification with Multi-Imprecise Labels},
school = {Univ. of Florida},
year = {2021},
address = {Gainesville, FL},
month = {April},
}