Deep neural networks are expressive functional templates to approximate unknown dynamics thanks to their universal approximation property. However, their expressiveness can also cause overfitting, especially when training samples are lacking. Our recent ACC ’22 paper deals with the issue by imposing physics-induced constraints (e.g., monotonicity and stability) on the learned dynamics. Such a method can suppress overfitting in learning complex chemical or biological systems.
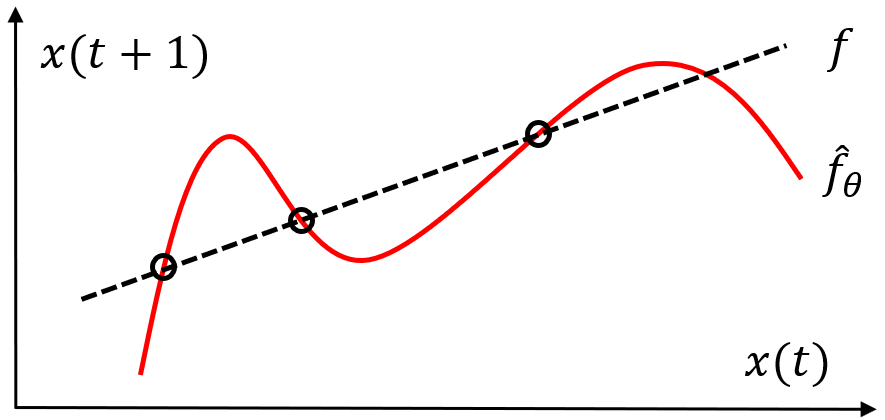